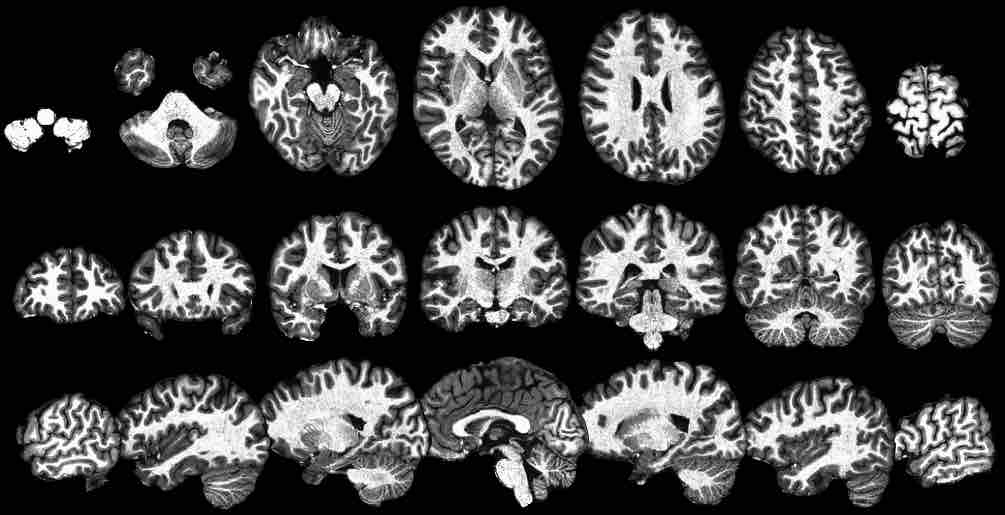
Reproducible scientific Python environments with conda
This is a brief explanation of a workflow that I’ve been using for research/data-science projects in Python. It makes use of conda
environments co-located with project files. This meets several key criteria that I was looking for:
- Environments are easily recreatable, meaning less worry about ever borking things
- Reproducible workspace across different machines
- Dependencies placed under version control for open-science and collaboration
- “Portable” environments that are easy to move around like normal folders
Below are the key steps to use this setup.
Note This post replaces an earlier version I was drafting using Craft. I took down that post from this site, but you can still access the draft version here. The commands below were run on macOS and should be relatively similar to other Nix-y systems (e.g. Ubuntu and Windows subsystem for Linux).
Setting up Anaconda or Miniconda
You can use this link to grab the latest Miniconda (on macOS you want the bash
script). Or you can use the installation the link at the bottom of this page to download Anaconda instead. Miniconda is a bit faster cause it’s more bare-bones, but Anaconda includes some default packages. Once you’ve downloaded either of those files you’ll need to open up a Terminal and cd
to the location of the file (probably Downloads
or Desktop
). From there you’ll need to run the installer and follow the prompts, which you can do by typing bash fileYouDownloaded.sh
.
You can verify the installation worked by asking your system what Python it sees now using which python
. If everything worked it should point to the Python installed inside your anaconda3
or miniconda3
directory.
Creating a new environment for each project
Most guides will tell you to create a new named environment using the -n/--name
flag to conda create
. But a more reproducible setup is to create a local environment within your project folder. Let’s say you have a project folder called myproject/
. The command below creates a new environment in a sub-directory called env
. It installs Python 3.8, pip
(for libraries on pypi), and specifically uses the conda-forge channel for grabbing them:
# From within myproject/
conda create -p ./env python=3.8 pip -c conda-forge
Change your Python version to what makes most sense for your project. You can also omit -c conda-forge
to just install from the normal defaults
channel.
You should now see a new env/
directory. You can activate the environment by pointing to it: conda activate ./env
. You should not commit this folder to version control as it can be quite large depending on how complex your project requirements get. So make sure to echo 'env/' >> .gitignore
.
Backing up and restoring your environment
This is the critical piece that makes this setup work: the environment.yml
file. This file is a recipe for rebuilding your environment in a platform-independent way. To create this recipe run the following command:
# Export the environment recipe to a file called environment.yml
conda env export --no-builds -f environment.yml
The --no-builds
flag exports the current environment in a platform-independent way. This means you should be able to use the same environment.yml
across different operating systems (e.g. macOS, Windows, etc)
You should rerun this command any time you install or uninstall new libraries and packages. You should also commit those changes to version control:
git add environment.yml
git commit -m "saved environment"
To restore an environment from this file (e.g. if you or a collaborator are working on another machine or you break something) just do:
# Make sure the env isn't active
conda deactivate
# Delete the env folder
rm -r env
# Create a new env using the spec in environment.yml
conda env create -p ./env -f environment.yml
You can also make sure your environment is in-sync with environment.yml
by running the following command which will install and uninstall dependencies as needed:
conda env update -p ./env -f environment.yml --prune
A few closing suggestions
Make sure you know what environment is active before running conda install commands
Make sure whenever you want to add or remove package to a project environment (e.g. myproject
), you conda activate ./env
it first. If you don’t, you’ll accidentally be adding and removing things from your base
environment!
Be careful about mixing and matching conda channels!
In snipped above I used the -c
flag to conda install
from the conda-forge
channel. By default conda
will install from the defaults
channel which points to anaconda.org, whereas conda-forge
points to conda-forge.org.
In general, you can save yourself a lot of headaches by simply sticking with the same channel for installing everything. For example if I installed numpy using conda install -c conda-forge numpy
, then it’s a good idea to keep using conda-forge
for other packages I want like pandas
: conda install -c conda-forge pandas
, rather than conda install pandas
, which is equivalent to conda install -c defaults pandas
.
There might be times when you can’t avoid mixing and matching, but it’s a good heuristic to help avoid the dreaded “environment conflict” messages that you might encounter otherwise. I have been a long time defaults
user because of one or two less than pleasant experiences with conda-forge
several years ago. Plus there used to be a significant speed difference when using the Intel compiled MKL (Math Kernel Libraries) and the open BLAS (Basic Linear Algebra Subprograms) that power libraries like numpy
. But lately most of that seems to have changed and for the last few projects I’ve been exclusively preferring conda-forge
for its breadth and especially for any R related dependencies.
Optional extra automation
If you’re interested in automating this workflow a bit, I made a few bash functions and aliases that you should be able to drop in to a .zshrc
or .bashrc
file. Specifically:
envinit()
for creating a brand newenv/
with a few basic libs and exporting itsenvironment.yml
envsave()
which exports the currentenv
toenvironment.yml
and also appendspip
packages installed from something other than pypi, e.g. github or locally from source. That’s because currently conda doesn’t install these packages when recreating an environment. So you’ll have to install them manually withpip
envcheck()
which checks ifenvsave
needs to be run and does so. Useful as a git pre-commit hook.envactivate()
which basically “overloads”conda activate
to prefer a./env
if one exists in the current directorynewproject.py
a python script that bootstraps a folder structure I often use while also setting up a VSCode Workspace and git repo
When starting a new project I’ll usually do something like this:
# Create new project scaffold using the `newproject` script at the end of this post
newproject --name coolscience
# Create a new conda env using an alias for the `envinit()` bash function
cd coolscience
ie
# Setup up verison control for env
echo 'env' >> .gitignore
git add environment.yml
git commit -m "saved environment"
This creates the following project structure and gives me a Python environment ready to go with some reasonable defaults and editor setup:
# contents of coolscience/
|-- analysis/
|-- code/
|-- data/
|-- docs/
|-- env/ # actual env contents
|-- figures/
|-- presentations/
|-- LICENSE
|-- README.md
|-- environment.yml # env recipe
|-- .vscode/
|-- .gitignore
As I continue working I’ll do the following:
- whenever I first
cd
intocoolscience
I’ll useca
to activate the environment installed incoolscience/env
- I’ll
ce
to check if myenvironment.yml
needs to be updated with any new packages Iconda install
-ed or removed - If I remember I’ll use
se
whenever Iconda install
something. But honestly I forget a lot so end up usingce
Note As mentioned above the environment.yml
generated by envsave()
and envcheck()
will also output installed pip
packages for convenience. However, any pip packages installed from source or after git clone
-ing something will need to be reinstalled manually whenever you recreate the environment. The aliases will print out a message indicating whenever you’re in that situation.
And the aliases/functions/scripts are here: